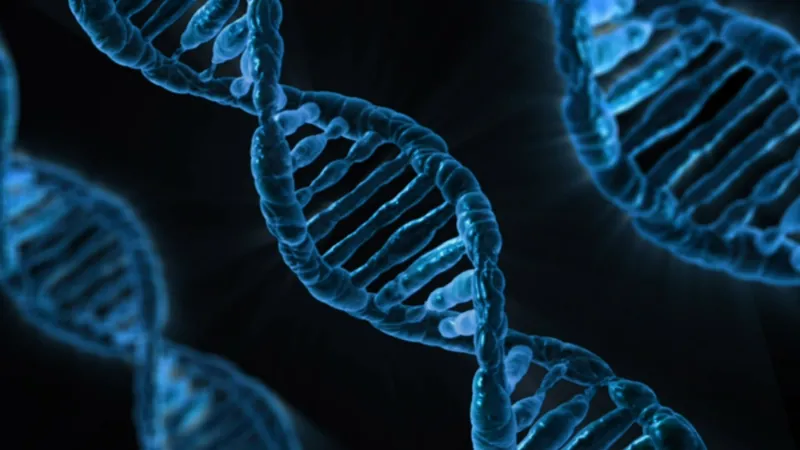
Groundbreaking Causal Theory Reveals New Pathways for Gene Targeting and Disease Treatment!
2024-11-07
Author: Jacob
Introduction
In a remarkable stride in genomic research, scientists at MIT have unveiled a pioneering causal theory aimed at deciphering complex relationships among genes. This innovative approach promises to revolutionize the way researchers understand gene interactions and could lead to targeted treatments for various diseases.
The Complexity of Human Biology
Human biology is astonishingly intricate, with approximately 20,000 genes capable of influencing one another in multifaceted manners. The challenge lies in the ability to identify which genes to focus on, as they operate in collaborative networks or modules that mutually regulate each other's activity. Until now, gathering the necessary data to infer these complicated relationships often demanded expensive and ethically questionable interventional experiments.
A New Theoretical Framework
However, the MIT team has developed a theoretical framework that utilizes only observational data—an approach that significantly reduces the need for costly experiments. By simply studying gene expression changes, researchers can unlock insights into cellular functions at a molecular level and improve understanding of disease progression.
Co-led by graduate student Jiaqi Zhang and master's student Ryan Welch, alongside senior author and professor Caroline Uhler, this research signifies a breakthrough in the application of causal disentanglement methods. Zhang commented, "Grasping the underlying mechanisms of cell states is crucial in genomics. By cleverly aggregating observed data, we can extract more interpretable and actionable insights."
Deciphering Gene Programs through Observational Data
The researchers aimed at developing a method to learn about "gene programs," which depict how clusters of genes coordinate their activities to influence biological processes like cell development. Traditional methodologies often relied on interventional data, which require active manipulations that can be impractical or unethical. Instead, the new methodology allows for effective insights using only observational data.
The innovative machine-learning algorithm designed by the researchers can synthesize related groups of gene variables without the need for experimental interventions. This method not only identifies causal modules but constructs a solid framework for understanding the intricate network of gene relationships. Uhler noted, "With a robust causal theory, our future efforts can refine this understanding for genetic applications and regulatory relationships."
Combinatorial Challenges and Layered Representations
The researchers employed advanced statistical techniques to compute the variance of gene variables, determining which ones hold significance in causal relationships. By systematically eliminating zero-variance variables, they were able to reconstruct an intricate layer-by-layer representation of gene interconnectivity. Zhang explained, "Deriving an efficient algorithm for this complex problem posed a significant challenge, but it was a critical milestone."
Their findings indicated that each variable embodies a collective group of genes, and the connections between these variables illustrate how these groups influence one another.
A Vision for the Future
Looking ahead, the MIT team plans to apply their breakthrough technique in real-world genetic contexts and explore scenarios where some interventional data might be accessible. This could enhance our understanding of gene networks and potentially lead to the identification of targeted drugs for treating specific diseases.
As researchers continue to unravel the mysteries of genetic regulation, this ground-breaking causal theory holds the promise of transforming modern medicine—ushering in a new era of personalized therapies aimed at treating complex conditions more effectively. Stay tuned, as more developments unfold in the quest for precision medicine!