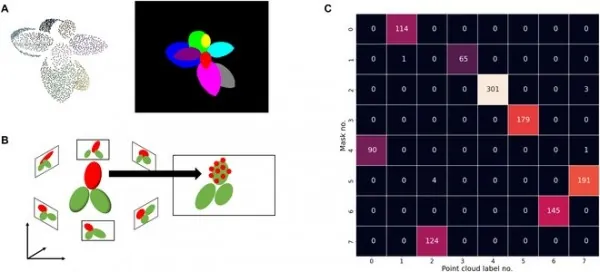
Groundbreaking 3D Reconstruction Method for Leaf Edges: A Game Changer in Agricultural Science
2024-11-11
Author: Michael
Introduction
A revolutionary new technique for 3D leaf edge reconstruction is set to transform our understanding of plant morphology, providing an unprecedented accuracy in analyzing leaf shapes essential for plant growth and agricultural optimization.
Importance of Leaf Morphology
Leaves play a vital role in photosynthesis and various physiological processes, showcasing a broad range of shapes that allow plants to adapt to their environmental conditions. Historically, the study of leaf morphology has depended on 2D imaging methods, which fall short in capturing the intricate 3D details necessary for comprehensive functional analysis of leaves. Although advanced 3D imaging techniques have emerged, mapping the edges of leaves, especially those with complex structures, continues to pose significant challenges.
The New Method
A recent study published in *Plant Phenomics* highlights a novel nondestructive method for leaf morphology analysis, offering a detailed look into plant structures in 3D. This innovative approach integrates deep-learning algorithms for 2D instance segmentation with curve-based 3D reconstruction, yielding remarkable results.
Testing and Results
The research team initially tested this method using virtual leaf models under optimal conditions. Here, 3D leaf edges were successfully reconstructed from 2D edge data derived from clear mask images. The performance of this technique was reliant on a support threshold parameter; lower values led to inaccuracies, while higher thresholds could result in incomplete reconstructions. When dealing with scenes featuring multiple leaves, accurately identifying which leaf corresponds to which edge was essential to overcome challenges posed by overlapping leaves.
The findings indicated that larger leaves were reconstructed with greater ease, whereas smaller leaves with more pronounced curvature presented complications. Although the method was affected by camera noise, it showed resilience to variations in the number of images or levels of occlusion; surprisingly, additional images did not always translate into improved accuracy.
Real-World Applications
Real-world applications were tested using actual soybean plants at different growth stages, where the method successfully reconstructed most of the leaves at preferred support thresholds. However, the technique struggled with small or heavily occluded leaves, occasionally producing artifacts or distorted shapes. Testing across various leaf types showed promising results, particularly with lobed edges, but highlighted a need for refinement when characterizing serrated and elongated leaves, especially at the apex. Notably, leaves featuring up to three holes were accurately reconstructed, though precision diminished as the number of holes increased.
Significance and Future Implications
Dr. Koji Noshita, the study's lead researcher, emphasized the significance of this advancement, stating, “Our method represents a breakthrough in 3D leaf edge reconstruction. By melding deep learning and curve-based methods, we can achieve a much greater detail in analyzing leaf morphology, which could have transformative implications for agricultural research.”
This innovative method not only marks a crucial step forward in plant phenotyping but also opens new doors for in-depth studies of plant morphology, influencing agricultural practices and ecological research. As this technology evolves, the researchers are optimistic that it will contribute to developing more effective agricultural strategies, ultimately addressing global food security issues.
Conclusion
In a rapidly changing world, advancements like these could prove invaluable in the quest for sustainable agricultural solutions. Stay tuned as this breakthrough continues to unfold, and prepare to witness the future of agricultural productivity redefined!