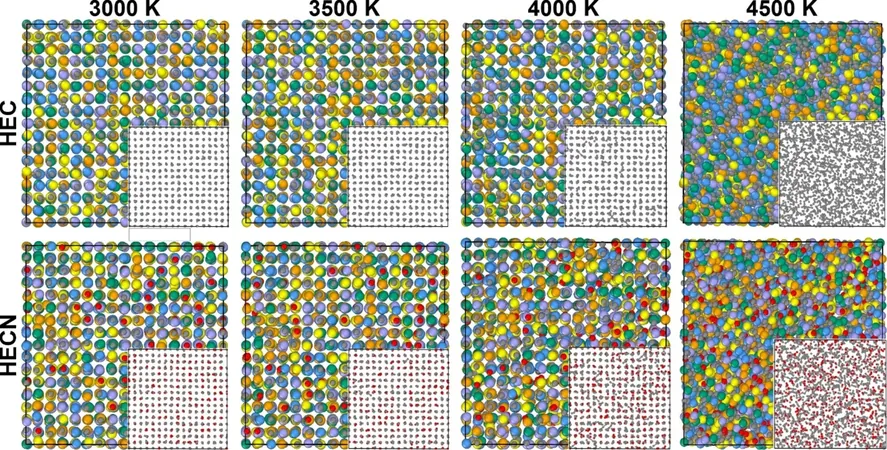
Breakthrough Research: Neural Networks Revolutionize High-Entropy Carbonitrides for Extreme Conditions!
2024-12-20
Author: Emma
Introduction
In a groundbreaking study conducted by a team at the Skolkovo Institute of Science and Technology (Skoltech), researchers have harnessed the power of neural networks to analyze the melting point of high-entropy carbonitrides—an advanced material composed of titanium, zirconium, tantalum, hafnium, and niobium, combined with carbon and nitrogen. This achievement not only promises to reshape our understanding of material science but also opens the door to new applications in environments characterized by intense heat, shock, and chemical exposure.
Importance of Melting Point Determination
Melting point determination is crucial for understanding materials' properties and their potential applications; however, traditional experimental methods can be both intricate and costly. The researchers at Skoltech tackled this issue by employing computational techniques that yield precise results in a fraction of the time and cost. Their findings have been published in the esteemed *Scientific Reports* journal, highlighting the significant role that high-entropy carbonitrides could play in protective coatings for extreme operational conditions.
Research Methodology
Professor Alexander Kvashnin, who oversaw the study, stated, “We utilized deep neural network-based potentials of interatomic interactions to model the structure of high-entropy carbonitrides, specifically TiZrTaHfNb)CxN1−x, in both solid and liquid states. This innovative approach enabled us to forecast heating and cooling temperatures related to varying nitrogen content, ultimately allowing us to calculate melting points and analyze the intricate relationship between structure and properties.”
Findings and Implications
Intriguingly, the researchers noted that increasing the nitrogen content within these compounds elevates the melting point, indicating a shift in the stability between the solid and liquid states. The Skoltech team also devised a novel methodology for training the DeepMD potential, effectively simulating melting and crystallization processes, which bolstered their ability to predict melting points with remarkable precision.
Advancements in Atomic Predictions
With atomic trajectories derived from ab initio molecular dynamics, this approach not only enhances the accuracy of atomic force and energy predictions but also expands upon traditional molecular dynamics modeling. This breakthrough holds implications beyond just high-entropy carbonitrides—it may transform how we model and understand a variety of complex, multicomponent materials.
Future Directions in Material Science
As industries continually seek materials capable of enduring extreme conditions, this cutting-edge research paves the way toward innovative solutions in high-performance applications, including aerospace, automotive, and energy sectors. The future of material science just got a powerful ally in neural network technology!