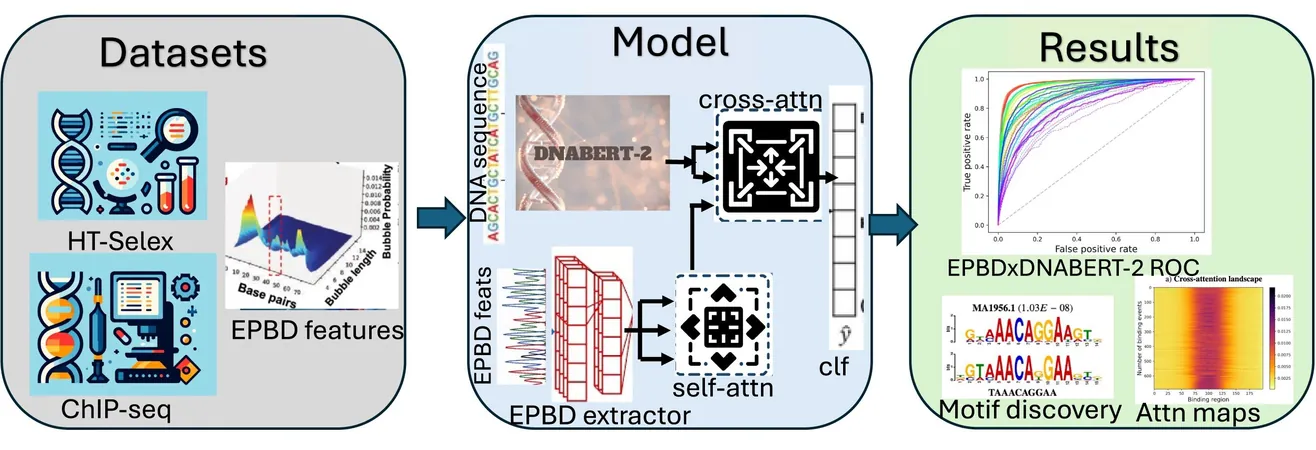
Breakthrough AI Model Revolutionizes Disease Gene Prediction
2024-11-11
Author: Liam
In a groundbreaking advancement for the field of genomics, scientists at Los Alamos National Laboratory have unveiled an innovative multimodal deep learning model named EPBDxDNABERT-2. This cutting-edge technology enhances the understanding of how DNA functions in relation to diseases, particularly by decoding the intricate relationships between transcription factors and the genomic landscape.
Transcription factors are vital proteins that play a key role in regulating gene expression—the process by which genes direct cellular function and development. Given the complexity of the human genome, which comprises approximately 3 billion base pairs, identifying how these transcription factors interact with specific locations on the DNA has been a formidable challenge. "The human genome is incomprehensibly large, and understanding which transcription factor binds to which part of this extensive genetic material is crucial for advancing disease treatment," stated Anowarul Kabir, the lead researcher.
Deep Learning Meets DNA Dynamics
The EPBDxDNABERT-2 model is a significant leap forward, employing a foundational algorithm specifically trained on DNA sequences and simulating various DNA dynamics, including an essential phenomenon known as DNA breathing. This process refers to the spontaneous opening and closing of the DNA double-helix, which can influence transcriptional activity and subsequent gene expression. By capturing these dynamics, the model enhances predictions about where transcription factors may bind within the DNA.
Manish Bhattarai, another key researcher in the project, elaborated, "Integrating the DNA breathing features with our foundational model has markedly improved the predictions of transcription factor-binding interactions." The model assesses sequences of DNA code to determine binding probabilities across different cell types, demonstrating a significant increase in accuracy—by 9.6%—in predicting the binding behavior of over 660 transcription factors.
Harnessing the Power of Supercomputing
For this ambitious project, the team utilized the Laboratory's advanced supercomputer, Venado, which combines powerful CPU and GPU capabilities to enhance AI processes. This state-of-the-art machine allows the model to identify complex patterns in the data, akin to the neural networks found in the human brain.
The training dataset for EPBDxDNABERT-2 comprised gene sequencing information from 690 experimental results that included 161 distinct transcription factors and 91 human cell types. This comprehensive approach also involved analyzing both controlled laboratory data and real-world datasets extracted from living organisms, such as mice, to ensure robust predictive capabilities.
Transforming Drug Development
The implications of this research are far-reaching. By improving the understanding of transcription factor interactions, EPBDxDNABERT-2 holds the potential to accelerate drug discovery and development, particularly for diseases linked to genetic regulation, such as cancer. The model's ability to extract binding motifs—the specific sequences that transcription factors target—further elucidates transcription mechanisms, providing invaluable insights for therapeutic interventions.
"Our multimodal foundational model demonstrates versatility and efficacy across various datasets, marking a significant advancement in computational genomics," said Bhattarai. This revolutionary tool not only enhances our understanding of complex biological systems but also paves the way for future innovations in medical research and treatment strategies, potentially transforming the landscape of genomic medicine.
Stay tuned as we continue to cover the exciting developments in AI and genomics, which are reshaping our understanding of health and disease!