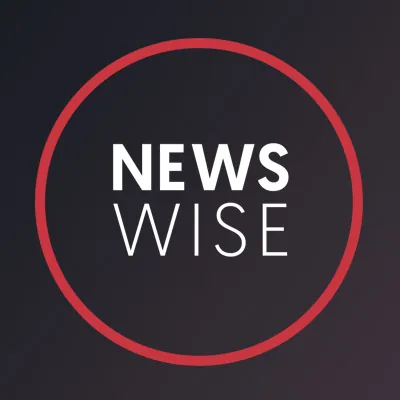
Alarming AI Limitations Uncovered by UW–Madison Researchers: Are Our Health Predictions in Jeopardy?
2024-11-04
Author: Emma
In a groundbreaking study, researchers from the University of Wisconsin–Madison are raising red flags about the increasing reliance on artificial intelligence (AI) tools in genetics and medicine. Their findings suggest that these tools can lead to erroneous conclusions regarding the connections between genes and physical traits, particularly in assessing the risks for diseases like diabetes.
The researchers delve into the complexities surrounding genome-wide association studies (GWAS), which analyze vast arrays of genetic variations across populations to uncover potential links between genes and different health conditions. While GWAS has been instrumental in revealing genetic influences on diseases, including the genetic basis of conditions like cystic fibrosis, the relationship between genetics and traits can be convoluted and nuanced.
The study sheds light on a significant challenge faced by researchers: many existing health databases, such as the National Institutes of Health’s All of Us project and the UK Biobank, often lack comprehensive data on the very diseases they aim to study. As noted by Qiongshi Lu, an associate professor at UW–Madison, the costs and labor involved in gathering thorough health data mean that many characteristics remain underrepresented, leading to limited samples.
To navigate this issue, researchers have increasingly turned to advanced AI and machine learning models to bridge data gaps and predict health-related traits. However, Lu warns that a lack of caution when using these models can lead to erroneous assumptions, highlighting a particular case where certain genetic variations were inaccurately linked to an increased risk of Type 2 diabetes.
If these machine learning predictions are accepted without careful scrutiny, they can result in “false positives.” These misleading results not only affect diabetes research but may permeate other areas of genetics, leading to significant errors in our understanding of genetic risks for various health conditions.
In response to these challenges, Lu and his team have proposed a new statistical method aimed at improving the reliability of AI-assisted GWAS. Their method is designed to combat bias introduced by machine learning algorithms when operating with incomplete data, thereby enhancing the accuracy of genetic associations in studies.
Moreover, the researchers have also highlighted potential pitfalls in studies utilizing proxy data—where available information, such as family health history, is used to infer risk for diseases with limited direct data, like Alzheimer's disease. Their findings indicate that such studies can yield misleading genetic correlations, particularly when attempting to connect Alzheimer’s risk with cognitive abilities.
As the team emphasizes, though genomic researchers often work with large biobank datasets, the statistical power gained from large sample sizes can amplify biases and increase the likelihood of errors. The cautionary message is clear: without rigorous statistical methodologies, the integrity of large-scale genetic research is jeopardized.
In an era where AI's influence continues to grow in health science, this study serves as a critical reminder to approach these advanced tools with a balanced perspective. As researchers navigate these complexities, ensuring the reliability of data and methodologies remains paramount in unveiling the intricate relationship between genetics and health. The implications of these findings could reshape the future of genetic research and its role in disease prediction.